Understanding Quartiles: Comprehensive Insights
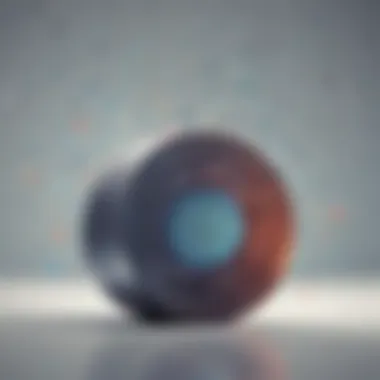
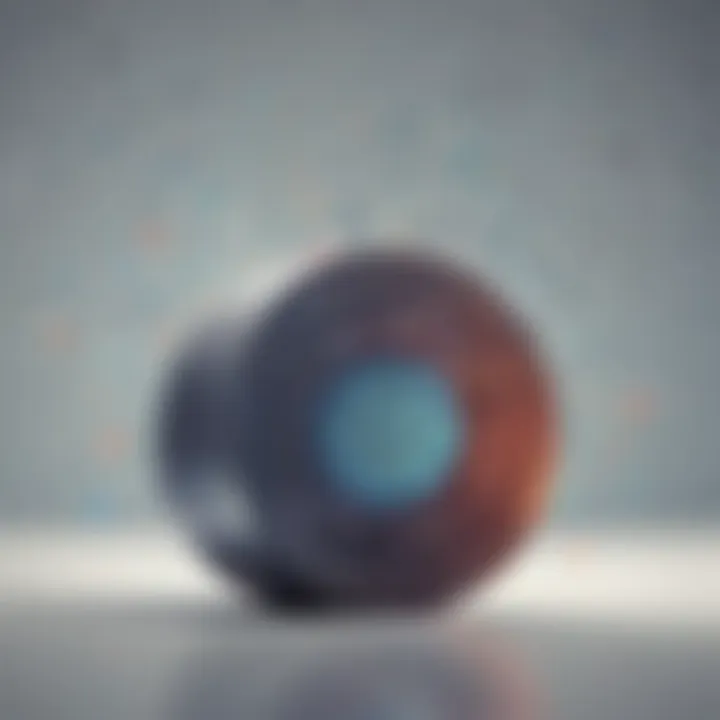
Intro
In the ever-evolving domain of data analysis, quartiles serve as crucial measurements that lend clarity to a multitude of data trends and variables. Unlike mere averages, quartiles split a dataset into four equal parts, illuminating how values distribute among the total observations. This methodology not only simplifies data interpretation but also digs deep into nuances that averages often gloss over. For anyone looking to enhance their financial literacy or embark on investment ventures, understanding quartiles offers a solid foundation for making informed decisions.
By delving into quarters of data, one can pinpoint outliers, identify medians, and ultimately grasp a more comprehensive narrative of the dataset. 'Knowledge is power,' but in the financial world, specificity is key. By the end of this article, you will understand how to calculate quartiles, their application in various sectors, and their significance in investment decision-making.
Investment Dictionaries
To fully appreciate the significance of quartiles, it is vital to get acquainted with some key terms and definitions that enhance our understanding of financial data analysis. This terminology is the backbone that supports the exploration of investment strategies and analytics.
Key Terms and Definitions
- Quartile: A quartile is a type of quantile that divides a ranked dataset into four equal parts. Each quartile represents a quarter of the data distribution.
- Median: The value that separates the higher half from the lower half of a data sample; the median is also considered the second quartile.
- Percentile: This divides data into one hundred equal parts, giving a more granular view compared to quartiles.
- Quintile: Similar to quartiles, quintiles split data into five segments, providing an additional layer of detail.
These terms are essential for anyone, especially investors, as they shape how data can be communicated and analyzed in various contexts.
Common Investment Strategies
Understanding quartiles isn't just academic; it can be applied practically in investment strategies. Investors can use quartiles to:
- Analyze stock performance by comparing a stock’s quarterly returns against its historical quartile distributions.
- Assess mutual funds in relation to their peers by evaluating their quartile rankings in terms of performance.
- Build diverse portfolios that minimize risk, as quartiles help identify volatile sectors versus stable ones.
By grasping these concepts, you'll find that there's more to investment decision-making than meets the eye.
"A smart investor learns to look beyond the numbers and understand the stories they tell."
Expert Advice
Whether you are just starting or you have a firm grasp on investment principles, quartiles can enrich your strategic approach.
Practical Tips for Novice Investors
For those who are new to investing, quartiles can help make sense of market trends:
- Start Small: Begin with investments in stocks or mutual funds that rank highly in the first quartile.
- Educate Yourself: Familiarize yourself with using quartiles for portfolio diversity—focus on stocks from different quartiles.
- Review Regularly: Keep tabs on your investments by evaluating their performance against quartile trends.
Advanced Techniques for Experienced Investors
For seasoned investors, quartiles provide a deeper analytical advantage:
- Trend Analysis: Use quartiles in conjunction with other data sets to forecast market movements.
- Risk Assessment: Identify which quartiles have historically been more volatile and adapt your portfolio accordingly.
- Performance Benchmarks: Set benchmarks based on quartile performance metrics to measure your investment's health over time.
By employing these methods, investors not only enhance their understanding but also sharpen their decision-making skills, ultimately leading to smarter, more strategic investments.
Intro to Quartiles
Understanding quartiles is like peeking into the soul of a dataset, and yet, many skim over this essential statistical concept. As we embark on this exploration, it's crucial to recognize the significance that quartiles bring to the table in various fields, especially for investors, analysts, and financial advisors. They offer not just mere numbers but insights that can steer decision-making processes, unveiling the true story behind the data.
Defining Quartiles
At its core, a quartile is a type of quantile that divides a dataset into four equal parts. Imagine throwing a dice; you can think of the four segments as the chances each side has to show up. The first quartile (Q1) represents the 25th percentile, meaning 25% of the data lies below this point. The second quartile (Q2), essentially the median, has 50% of the data below it. Then there’s the third quartile (Q3) at the 75th percentile, below which 75% of the readings fall. Finally, data above Q3 is where the fourth quartile lies, encompassing the top 25% of values.
Calculating quartiles might seem daunting, but it’s fairly straightforward. Take your dataset, organize it, and simply find the values at these critical markers. This method proves useful for gauging the variability and spread within your dataset, providing context that raw numbers alone often lack.
The Importance of Quartiles in Statistics
Quartiles serve as a foundational pillar in statistics, offering clarity and perspective often obscured in standard mean or median calculations. Here’s why they matter:
- Insight into Distribution: Quartiles break down data points into meaningful segments, helping illustrate ranges and pinpoint disparities.
- Identifying Outliers: By understanding where most data clusters, one can easily spot potential outliers. This is particularly crucial in finance, where outlier data could signal risk.
- Decision-Making: Investors often rely on quartile rankings for assets or securities to compare performance. Knowing whether an investment rests in the top or bottom quartile informs strategies and resource allocation.
In summary, quartiles do more than just split the dataset; they provide a framework to interpret and analyze data effectively. When employed properly, they can enhance the decision-making process by offering a more nuanced view.
"In finance and statistics, anything that gives a clear picture can be the difference between success and blunders.*"
Understanding these nuanced relationships between different quartiles can pave the way for smarter investing and better analyses, making grasping this concept imperative for anyone seeking to deepen their understanding of statistical measures.
Calculating Quartiles
Knowing how to compute quartiles is like having a compass in unfamiliar territory. It directs investors and analysts toward better decision-making by illustrating data distribution and variance signified by these critical values. Less about crunching numbers and more about interpreting trends, calculating quartiles can expose hidden insights and make the invisible visible. Moreover, it supports multiple applications, from assessing risks in investments to pinpointing student performance in academic scenarios.
Step-by-Step Calculation
To calculate quartiles, follow these straightforward steps:
- Arrange Your Data: Start by sorting your data set in ascending order. This step is crucial because the quartile values are dependent on the order.
- Determine the Median: The second quartile (Q2) is simply the median of the data set. If you have an odd number of observations, the median is the middle number; if even, it is the average of the two middle numbers.
- Identify Q1 and Q3: The first quartile (Q1) is the median of the lower half of the data (the points below Q2), while the third quartile (Q3) is the median of the upper half.
- Formulate Quartiles: After step three, your quartiles will provide you with insights into how your data is spread out:
- Calculate the Interquartile Range (IQR): The IQR is simply Q3 - Q1. This indicator depicts how spread out the middle 50% of the data is. A wider IQR points to greater variability.

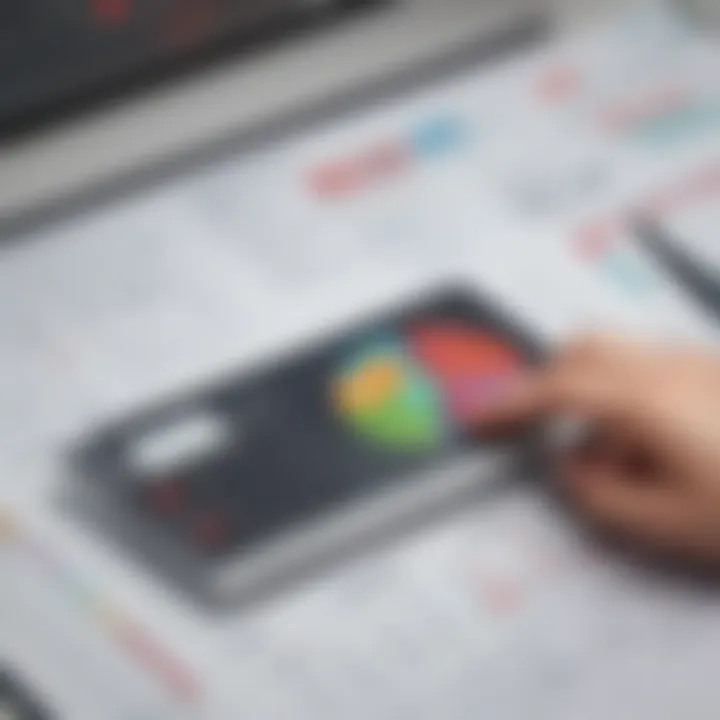
- Q1 divides the lowest 25% of data points.
- Q2 gives the overall median (50%).
- Q3 highlights the lowest 75% of data points.
These steps form the cornerstone of quartile calculation, enabling users to achieve detailed statistical clarity.
Tools for Calculation
When it comes to calculating quartiles, utilizing the right tools can dramatically enhance efficiency and accuracy. Here’s a look at some commonly used options:
Statistical Software
Statistical software, like R or SPSS, plays a pivotal role in quartile calculations. These programs can handle hefty data sets, making them ideal for complex analyses. One of the standout features is their ability to visually represent data, helping users to quickly grasp the results.
- Key Characteristic: The ability to automate repetitive tasks eliminates human error and saves time.
- Benefit: Particularly suited for advanced users, statistical software can manage datasets of varying sizes and complexities, offering in-depth statistical analysis.
- Unique Feature: R, for instance, provides built-in functions specifically tailored to compute quartiles, hence streamlining the process further.
Nevertheless, while these tools are powerful, they can come with a steep learning curve, potentially posing a barrier for less experienced users.
Spreadsheets
Spreadsheets like Microsoft Excel or Google Sheets are another common avenue for quartile calculation. They are highly accessible and user-friendly, catering to analysts across skill levels from novice to expert.
- Key Characteristic: Their intuitive interface allows users to visualize data quickly, which enhances understanding.
- Benefit: Nearly every professional has access to spreadsheet tools, making them a practical choice for everyday calculations.
- Unique Feature: A handy built-in function (like in Excel) makes calculating quartiles as simple as clicking a button, thus facilitating quicker analyses.
However, while they are versatile, spreadsheets might struggle with very large datasets, where performance can lag.
Getting comfy with these tools and knowing when to use one over the other can be your ace in the hole, empowering you to make better-informed decisions efficiently.
Practical Examples of Quartiles
Understanding quartiles is not just an academic exercise; it has real-world implications across various arenas, particularly in finance and education. In this section, we will unwrap practical examples that showcase the importance of quartiles and their effectiveness in analyzing data. These examples illustrate how quartiles can guide decision-making and highlight disparities within datasets.
Example in Investment Analysis
Investment analysis serves as a prime illustration of quartile applications. Investors often rely on quartiles to assess the performance of various assets and portfolios. This helps highlight trends, risks, and rewards that might not be immediately apparent.
Analyzing Investment Returns
When it comes to analyzing investment returns, quartiles provide a clear framework. By categorizing returns into four distinct groups, an investor can evaluate where their investment stands relative to market averages. For instance, if a portfolio falls into the bottom quartile, it indicates underperformance compared to 75% of the investments in the same category.
This characteristic makes analyzing investment returns using quartiles a favored method for many seasoned investors. It allows for an immediate grasp of performance with less heavy lifting.
However, one of the unique features of this analysis is that while it simplifies performance comparison, it can obscure individual performance nuances. An investment returning 10% might seem appealing, yet if the top quartile is yielding 25%, that 10% becomes less impressive in context.
Difference Between Top and Bottom Quartiles
The comparison between the top and bottom quartiles is vital in investment contexts. This contrast helps investors draw insights about risk and reward across different investment products. Understanding this difference can shape investment strategies and lead to more informed choices.
The key characteristic of looking at these two quartiles is the immediate assessment of risk. For example, the bottom quartile's assets might be experiencing volatility or downturns while the top quartile shows stability and growth.
This approach offers a beneficial path to seeing where the strongest market movers are and why they perform well. However, it's essential to appreciate that a heavy focus on top quartiles might lead to overlooking potentially lucrative investments in the lower quartiles that could have growth potential.
Example in Academic Scoring
Quartiles are equally significant in the realm of education, where they help highlight student performance levels. Educators and administrators employ quartile analysis to gain insights into how students are performing relative to their peers.
Distribution of Student Performance
In examining the distribution of student performance, quartiles can paint a telling picture of academic achievement. Schools often categorize student scores into quartiles, allowing them to analyze the effectiveness of their teaching methods and recognize trends over time.
This practice helps identify areas where students struggle while also highlighting the high achievers. By concentrating on quartiles, schools can devise strategies to help bolster the performance of those in the lower quartile while continuing to challenge and enrich the higher-performing students.
It’s worth noting that while this distribution is helpful for assessing overall performance, there can be drawbacks. Schools might mistakenly channel all resources toward the bottom quartile without considering individual student needs.
Identifying At-Risk Students
Identifying at-risk students is another crucial application of quartiles in education. By focusing on those falling within the lower quartile, educators can deploy targeted interventions to assist struggling learners, potentially changing their academic trajectory.
This characteristic of quartile analysis is particularly beneficial—by highlighting at-risk students, schools can ensure they allocate support and resources effectively. With the right strategies in place, the chances to improve student outcomes are enhanced dramatically.
However, one has to approach this method carefully. Too much focus on categorization can lead to stigma or discourage students from attempting to improve their academic standing due to a label they might not agree with.
These examples showcase quartiles as powerful tools in analyzing both financial data and educational performance. They help inform decisions, shape strategies, and optimize resource allocation in diverse fields.
Quartiles versus Other Statistical Measures
Understanding how quartiles relate to other statistical measures is pivotal for investors and consultants relying on data to inform their decision-making processes. Quartiles are specifically designed to slice a dataset into four equal parts, providing valuable insight into distribution patterns. Compared to other statistical measures, quartiles illuminate different facets of data, such as spread and central tendency. Notably, they manage to condense large volumes of data while still highlighting significant relationships within it.
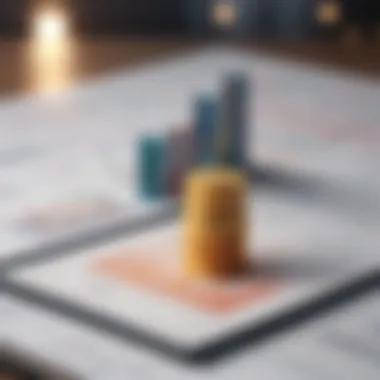
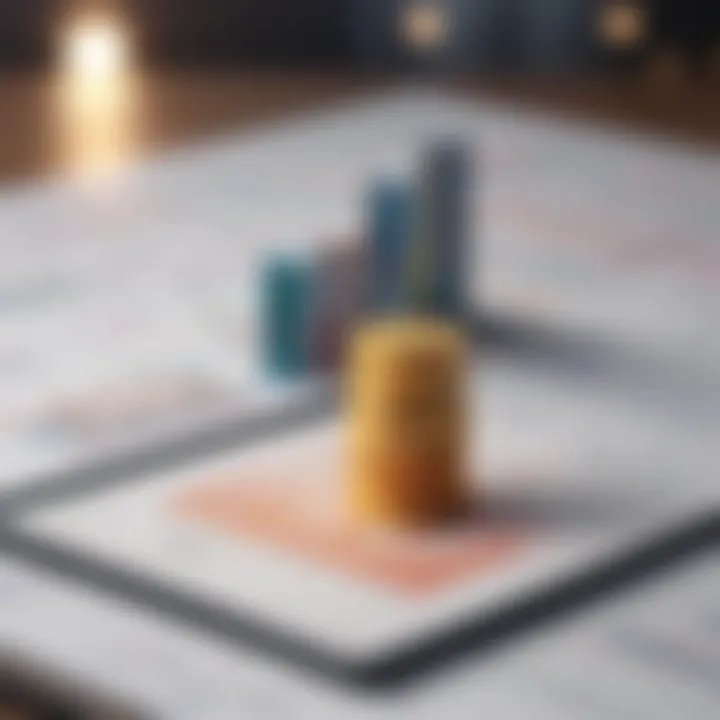
When making analyses, understanding the distinction between quartiles, quintiles, and percentiles becomes crucial. Each of these measures serves a unique function, tailoring the analysis to the needs of the user or the context of the data.
Comparing Quartiles and Quintiles
To kick things off, let’s discuss quartiles and quintiles. While quartiles divide a dataset into four equal segments, quintiles do so into five. For example, imagine a group of students' scores in a standardized test:
- Quartiles would break down these scores into segments like the top 25%, next 25%, and so forth.
- Quintiles would take it a step further by adding another category, creating five distinct groups.
This additional slice in quintiles enables analysts to gain a more granular understanding of the distribution. For investors, analyzing data through quintiles can provide comprehensive insights into performance metrics and risk factors. However, it may also introduce increased complexity, as the additional divisions might obscure broader trends that quartiles efficiently capture.
Understanding the Differences Between Quartiles and Percentiles
Then there’s the topic of percentiles. Percentiles stand apart from both quartiles and quintiles. They break a dataset into 100 equal parts, allowing for remarkably detailed insights. To illustrate:
- If someone scores in the 80th percentile on a test, they performed better than 80% of the participants. Meanwhile, if they land in the second quartile, they are in the 50-75% range, meaning their performance is better than half but not quite reaching the excellence of the best quarter.
Understanding this difference is substantial for applications in finance. For instance, investors often use percentiles to assess relative performance in investment portfolios. This level of detail within percentiles may prove beneficial in identifying nuanced performance but can overwhelm those unaccustomed to navigating large datasets.
For effective data analysis, knowing whether to utilize quartiles, quintiles, or percentiles can hinge on context. Each measure brings its own set of insights, offering unique benefits and considerations.
In closing, grasping how quartiles stack against other statistical measures enriches one's data interpretation skills, ultimately refining investment strategies and informing more accurate decisions.
Applications of Quartiles in Finance
When we talk about finance, quartiles are not just some numbers on a piece of paper; they're the lifeblood of data analysis. They help investors and analysts make sense of vast amounts of data by breaking it down into manageable segments, allowing for a clear picture of performance and risk. Understanding how quartiles function within a financial context can provide insights into different investment strategies and the behavior of various financial metrics.
The world of dollars and cents can get overwhelming. But by implementing quartiles, investors can compare and contrast performance across different sectors or asset classes, dive into individual stocks, and really hone in on the top and bottom performers. It doesn't stop there; these number slices can guide decisions on diversification and risk management, making them a pivotal part of the investing toolkit.
Risk Assessment Using Quartiles
In financial decision-making, risk is always lurking in the shadows. Handling it effectively can be the difference between crippling losses and successful investments. Quartiles come into play by allowing investors to categorize investment risks in a structured way. By examining how investments perform across different quartiles, analysts can pinpoint which investments lie within the risky end and which ones are basking in stability.
For instance, by evaluating the upper and lower quartiles of financial metrics, like return on investment or volatility, analysts can gauge where the majority of their investments fall. They can see if they are disproportionately exposed to high-risk assets, and that awareness can guide them to reallocate their portfolios accordingly.
The ability to visualize data means less guesswork, and quartiles provide that clarity so essential for sound investment practices. They enable investors to say, "Hey, my risk is concentrated in the lower-performing assets," allowing for timely adjustments.
Investor Behavior Analysis
Understanding how different investors behave can reveal patterns that predict market movements. By segmenting investments into quartiles based on investor profiles, market analysts can observe trends that emerge with different groups of investors.
Behavior of Different Investor Profiles
Consider this: not all investors think alike. Some folks, like seasoned professionals, may take on high risks for the potential of high returns. On the other hand, conservative individuals might lean towards low-risk investments. This diversity results in different behaviors when it comes to key financial decisions.
The key characteristic of assessing investor profiles is that it provides a nuanced lens through which to view market fluctuations. For instance, high-risk tolerance investors might dominate the upper quartiles, frequently changing their investments to chase opportunities. Conversely, risk-averse investors often find their investments firmly nestled in the lower quartiles, seeking steady and predictable growth.
From this, one can draw advantages like predicting market trends or gauging when to act. But be careful; while identifying these behaviors can guide capital allocation and strategic decisions, it may also lead to over-generalizations if one isn't careful.
Strategies Based on Quartile Analysis
Finally, let's get down to the nitty-gritty of strategies based on quartile analysis. These strategies can revolve around how investors position themselves within the quartiles of potential returns. Investors can craft portfolios to allocate more to assets that consistently perform in the higher quartiles, maximizing return potential.
A unique feature of this strategy lies in its potential for increased gains without taking significant risk. Growth-oriented investments tend to overlay in the upper quartiles, where the payouts can be substantial. However, the challenge here is identifying which investments are worth it.
Embracing quartile analysis can also highlight underperforming assets, providing signals for divestment. However, it requires precise navigation of data and a thick skin against emotional investing decisions.
To wrap it up, quartiles serve as a compass in the ever-shifting financial landscape. They simplify the complex, enabling investors to assess risk and behavior effectively. And while they provide valuable insights into strategic approaches, they also come with their own considerations and risks, something that every astute investor ought to bear in mind.
Limitations and Misinterpretations of Quartiles
Understanding the limitations and potential misinterpretations of quartiles is crucial for anyone relying on this statistical measure in their analysis. While quartiles are valuable tools for summarizing data dispersions, they come with their own set of challenges. Investors, financial advisors, and analysts must be aware of these aspects to avoid pitfalls that could lead to misguided conclusions.
Common Pitfalls in Quartile Analysis
When engaged in quartile analysis, one can easily trip over common misconceptions that may distort the true picture of data distributions. Here are some notable pitfalls:
- Over-reliance on Quartiles Alone: Some individuals tend to focus solely on quartiles for understanding data distribution, neglecting other important measures like median, mean, or standard deviation. This perspective can yield a simplified understanding of complex datasets.
- Misinterpreting Quartile Ranges: The boundaries of each quartile clarify central tendency and variability. Misreading these ranges can lead to incorrect assumptions about data points falling into specific categories.
- Ignoring Sample Size: Smaller datasets can skew quartile results, making them less reliable. For example, in a dataset with limited observations, a single extreme value can disproportionately affect quartile calculations.
- Assuming Normal Distribution: Quartiles provide insight into data spread regardless of its distribution form. Relying on the assumption that the dataset adheres to a normal distribution can lead to overestimating the stability of these quartile measures.
In light of these pitfalls, it’s imperative to approach quartile analysis with a comprehensive strategy, incorporating multiple statistical methods to gain a well-rounded view of data.
Addressing Misinterpretation Issues
Misinterpretation of quartiles is not uncommon and can result from a variety of factors. To address these challenges, one can adopt specific measures to mitigate misunderstanding:
- Clear Definition of Data Context: Before diving into quartile analysis, it's essential to definitively understand the context and the dataset. What does it represent? Given context clarifies interpretations.
- Supplemental Statistical Measures: Pairing quartile data with other statistical tools, like histograms or box plots, can visually represent the distribution, making it easier to interpret quartile placement accurately.
- Training and Education: Organizing workshops or education sessions on quartile interpretation can enhance skills, leading to fewer misinterpretations within your team.
- Frequent Data Review: Regularly revisiting the data and its quartile distribution can lead to better understanding. Time can change the data landscape, so current information is vital.
- Consultation with Experts: When in doubt, consulting statisticians or analysts can help clarify complex issues arising from quartile calculations.
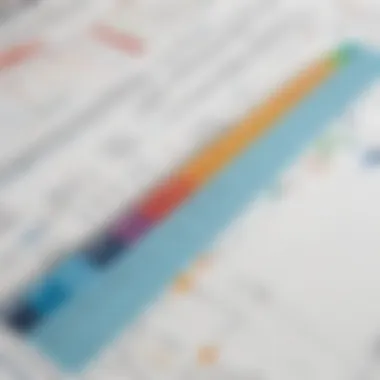
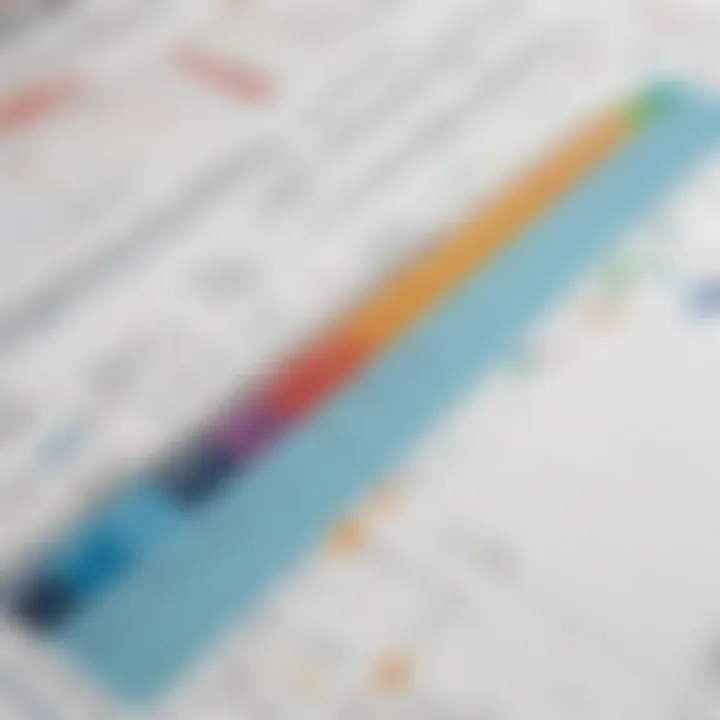
By addressing misinterpretation issues with these strategies, financial professionals can better leverage quartiles to inform decisions and analyses. Ultimately, a cautious and informed approach is necessary to maximize the utility of quartile data.
Case Studies Involving Quartiles
Analyzing quartiles through case studies can illuminate their practical application and effectiveness in real-world scenarios. Using quartiles allows decision-makers in various fields to break down complex data and gain valuable insights. The identified patterns can lead to better strategies, ultimately benefiting those who rely on statistical data for their decisions. These case studies serve as practical illustrations of how quartiles can influence both marketing strategies and healthcare analytics, showcasing the significance of this statistical concept.
Case Study in Retail Marketing
Identifying Target Markets
Identifying target markets is a crucial process for any retail business looking to maximize their revenue potential. By employing quartiles to segment customer data, companies can pinpoint which categories of customers are most profitable. For instance, if a clothing retailer uses quartiles to analyze purchasing behavior, they may find that a certain 25% of customers consistently buy luxury items.
The key characteristic of using quartiles in this context is its ability to reveal patterns among different demographic segments. When businesses know which groups spend more, they can tailor marketing efforts more effectively. This method acts as a compass guiding marketing strategies, ensuring that resources are directed toward tapping into those lucrative demographics. It’s a beneficial choice because it reduces guesswork, allowing for data-driven decisions.
While quartiles streamline the process of understanding consumer behavior, one downside is that they may oversimplify diverse client bases. Failing to consider the nuances within quartile groups could lead to missed opportunities or misinterpretations.
Sales Performance Segmentation
Sales performance segmentation leverages quartile analysis to categorize sales teams based on effectiveness and output. By ranking team performance into quartiles, managers can focus on top performers while also identifying those who may need further training. For instance, if 25% of the sales team consistently exceeds their targets, that group can be studied to extract best practices.
The unique feature of this approach is the clarity it brings to performance evaluations. Highlighting high achievers helps boost morale and creates a competitive environment. By emphasizing the top quartile, businesses not only identify what works but can instill a culture of success throughout the company.
However, while this method incentivizes high performance, it can create undue pressure on lower-performing teams. Retaining a balance is important; a too rigid focus on quartiles might diminish morale among those who are not performing at the same level.
Case Study in Healthcare Analytics
Patient Outcome Analysis
In healthcare analytics, quartiles can be instrumental in evaluating patient outcomes. By reviewing patient datasets, healthcare providers can categorize outcomes into quartiles to better understand trends related to treatment success across different patient groups. For example, if patients receiving a new treatment are grouped into quartiles based on recovery time, hospitals can identify what methods yield the best results quickly.
The significant advantage here lies in its implications for improving patient care. It allows practitioners to refine treatment protocols based on statistical evidence, thereby enhancing service delivery. Understanding patient outcomes through quartile analysis can highlight areas where healthcare can improve.
However, focusing exclusively on quartiles might mask important individual cases that do not follow the general trends. Healthcare providers should remain cautious and not overlook valuable insights that lie outside the quartile edges.
Resource Allocation Decisions
Resource allocation decisions represent another engaging application of quartile analysis in healthcare. By using quartiles to categorize resource utilization—whether it relates to staffing, equipment, or patient care—healthcare administrators can make more informed decisions. For instance, evaluating which quartile of departments uses the most surgical resources allows for targeted boosting of departmental budgets to meet demands.
The distinct feature of quartile use here is its capability to reveal imbalances in resource allocation. When administrators use quartiles, they can ensure that resources are distributed based on need rather than perception, ultimately aiming for a more equitable and efficient system.
On the flip side, relying solely on quartile analysis might lead to oversights where certain departments might have unique needs that get drowned out by the overall analysis. It’s crucial to blend both quantitative and qualitative assessments for optimal decision-making.
Best Practices for Using Quartiles
When it comes to employing quartiles in data analysis, understanding best practices is crucial for yielding reliable insights. Knowing how to effectively use quartile data not only enhances the clarity of your analysis but also boosts decision-making prowess in various fields, especially finance. By adhering to best practices, investors and analysts can minimize errors and ensure their conclusions are based on sound statistical reasoning.
Data Preparation Techniques
Adequate preparation of data is the backbone of any analysis involving quartiles. Getting it right at this stage can save analysts a world of trouble down the line. Here are some key techniques to consider:
- Ensure Proper Data Collection: This is about capturing accurate and relevant data. Double-check sources and methods of collection. Mismatched information can skew quartile divisions.
- Clean the Dataset: Often overlooked, this step entails removing duplicate entries, addressing missing values, and handling outliers. For instance, outliers can disproportionately affect the quartiles, leading to misleading interpretations.
- Standardize Formats: Consistency in data formats—dates, currency, units—can prevent confusion and enhance comparability. A dataset mixed with different date formats can complicate calculations and interpretations.
- Utilize Software Tools: Employ tools like R or Python to handle data preprocessing. They offer efficiency and precision in managing large datasets. A little code snippet like the one below can filter out unnecessary values effectively:
- Contextualize Your Data: Understanding the context around your dataset is also vital. If certain demographics or time frames are more relevant than others, focusing on those subsets can provide a clearer picture of where quartiles stand.
By following these preparation techniques, analysts lay a solid foundation for a refined quartile analysis.
Effective Interpretation Strategies
Once the quartiles have been calculated and insights drawn from data, the next step is to interpret the findings accurately. Misinterpretation can lead to faulty conclusions, which can cost both time and financial resources. Here are several strategies that can sharpen your interpretation skills:
- Contextual Comparison: Rather than looking at quartiles in isolation, compare them with historical data or benchmarks. This could involve analyzing shifts from lower to upper quartiles over several years.
- Visual Representation: Using box plots or histograms can vividly present quartile data. A visual representation often illuminates trends and disparities that mere numbers might obscure.
- Understand Limitations: Be mindful of the constraints associated with quartiles. For instance, quartiles summarize data but may overlook underlying issues such as skewness, which affects how data points are distributed in relation to the quartiles.
- Add Qualitative Insight: Blend quantitative results with qualitative assessments. If dealing with investment returns, input from market analysts can give more context to why particular quartiles behave as they do under fluctuating conditions.
- Stakeholder Communication: Present your findings in a manner that caters to your audience, be it financial advisors or investors. Tailoring the complexity of your explanation to the audience's level of understanding can foster better discussions and decisions.
In summary, the marriage of meticulous data preparation and astute interpretation strategies paves the way for proficient quartile analysis. Following these best practices equips financial professionals to leverage data effectively, ultimately informing their strategic choices.
Future Trends in Quartile Analysis
In today's data-driven world, quartile analysis is taking on new dimensions. The importance of this topic cannot be overstated, especially as the sheer volume and complexity of data increase. Investors, financial advisors, and analysts are seeking enhanced methods to gain insights, and quartiles fit snugly into this growing need. They help slice and dice vast datasets to highlight significant patterns and trends. Understanding the future trends in quartile analysis can equip these professionals with tools that transcend basic interpretations.
Rising Importance in Big Data
As businesses worldwide embrace big data, the quantity of information available can be overwhelming. This is where quartiles shine, helping to break data into manageable pieces. Let’s break down why quartiles are becoming more essential in big data analytics:
- Enhanced Decision-Making: By segmenting data into quartiles, analysts can pinpoint trends that might be missed in traditional reports. For instance, a retail company can identify which sales figures fall into the top quartile and which do not, informing marketing strategies.
- Focused Analysis: In big data, it is crucial to not just analyze data but to understand what it means. Quartiles allow analysts to focus on specific segments — like the lowest and highest-performing products — providing insights that are immediately actionable.
- Identification of Outliers: Quartile analysis helps in identifying outliers effectively. For instance, if a product is performing well above the top quartile range, it might mean it's time to expand the product line or focus marketing efforts. Conversely, items in the lower quartile might signal areas needing improvement.
"With data growing like weeds, quartiles provide the necessary clarity in the midst of confusion."
Integration with Machine Learning Techniques
Machine learning is rapidly becoming a game-changer in data analysis, and quartiles can be a part of this evolution. Here we explore how the merging of quartiles and machine learning is paving the way for superior data understanding:
- Feature Engineering: Machine learning algorithms rely significantly on the features fed into them. Quartiles can play a role in creating meaningful features. For example, by coding categorical data based on quartile performance, a model can achieve better accuracy.
- Model Evaluation: Analysts can segment predictions or outcomes into quartiles for enhanced evaluation. Evaluating model performance over quartiles can reveal how well a model predicts across different levels of an outcome, such as sales performance.
- Bias Detection: In machine learning, bias can creep into models unnoticed. By using quartiles, developers can analyze how different quartiles fare in predictions. If lower quartiles consistently yield inaccurate predictions, this may highlight a bias in the model that needs addressing.
In summary, as the digital landscape continues to evolve, integrating quartile analysis with big data and machine learning not only enhances the decision-making process but also provides a robust framework for comprehensively understanding datasets. For investors and analysts, keeping an eye on these trends is not just beneficial; it has become imperative.